Retrieval-Augmented Generation: The Future of AI Language Models
Zensark AI Division
July 16, 2024
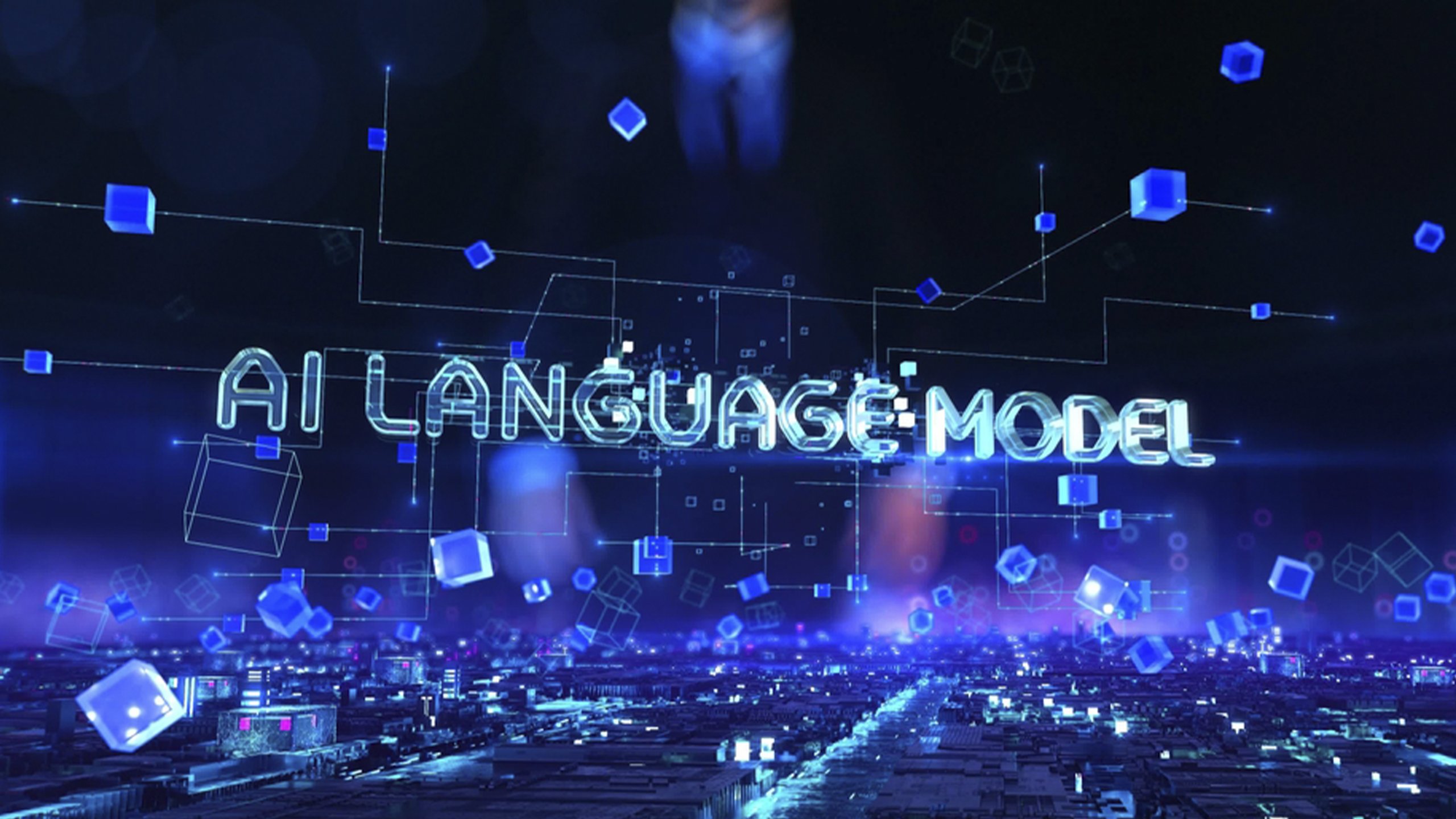
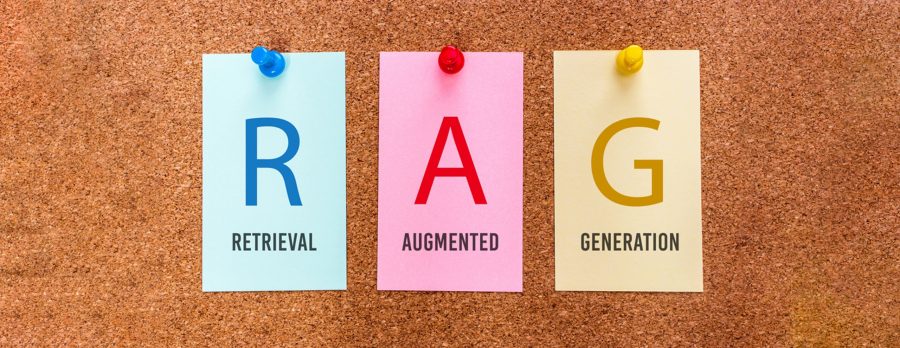
What is Retrieval-Augmented Generation?
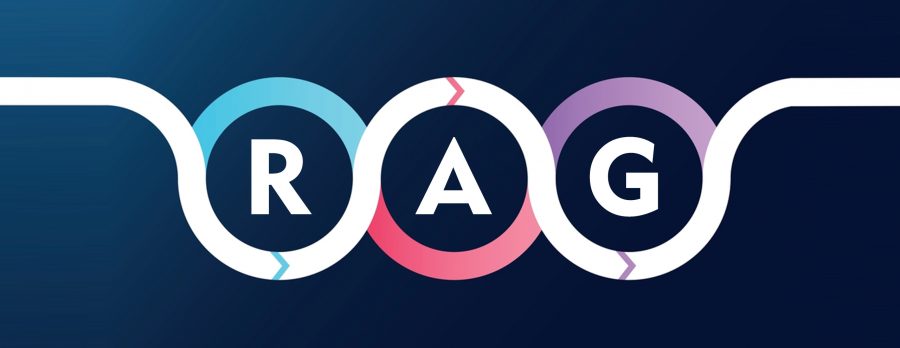
How Does RAG Work?
- Query Phase: When you input a question or prompt, the system searches through vast knowledge sources to find relevant information.
- Retrieval Phase: The system then selects the most pertinent passages or documents from the search results.
- Generation Phase: Finally, using both the original query and the retrieved information, the AI generates a response that is more contextually relevant and factually accurate.
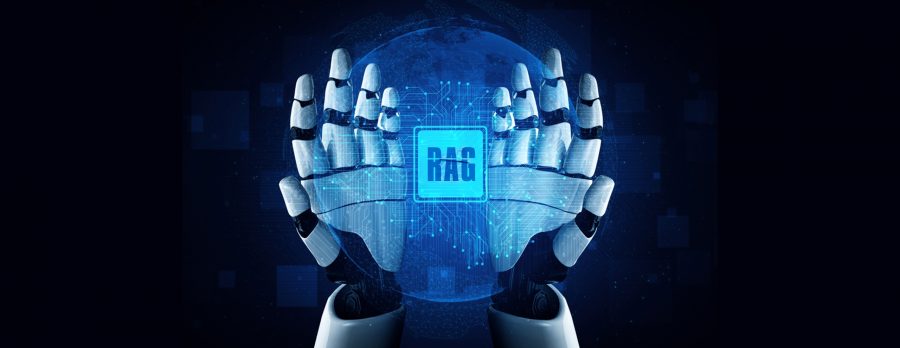
The Benefits of RAG
- Reduced Bias: By pulling from diverse sources, RAG helps mitigate biases that might be present in a single dataset.
- Fewer “Hallucinations”: RAG significantly reduces the risk of AI generating false or nonsensical information by grounding responses in real-world data.
- Improved Quality: The combination of retrieval and generation techniques leads to more relevant, fluent, and coherent responses.
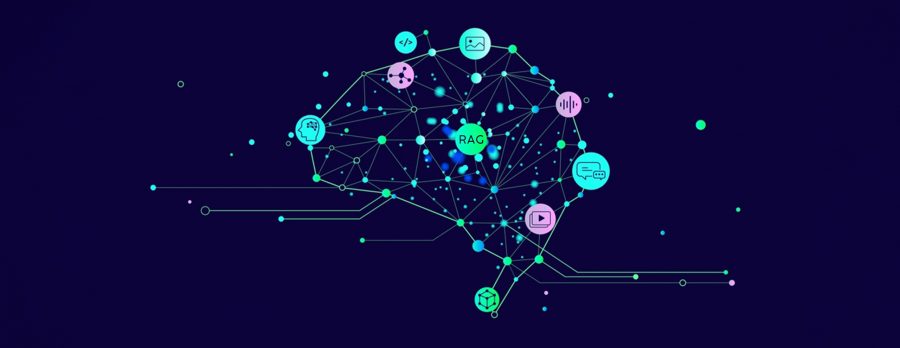
RAG vs. Fine-Tuning: When to Use Which?
While both RAG and fine-tuning have their places in AI development, they serve different purposes. RAG excels when tasks require access to external knowledge and benefit from contextual understanding. It’s ideal for applications like question-answering systems, dialogue models, and content generation.
Fine-tuning, on the other hand, is better suited for specialized tasks within a specific domain, such as sentiment analysis or named entity recognition. It offers faster response times but may lack the breadth of knowledge that RAG provides.
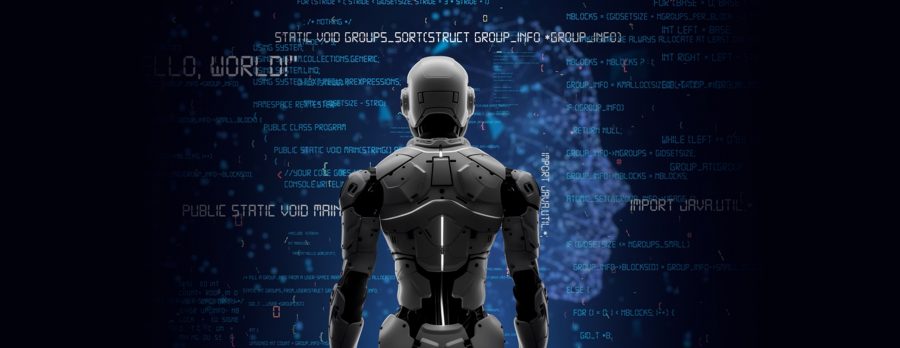
Real-World Applications of RAG
- Advanced Question-Answering Systems: Imagine customer support chatbots that can pull from a company’s entire knowledge base to answer queries.
- Enhanced Search Engines: RAG can help search engines provide more contextually relevant results, going beyond simple keyword matching.
- Powerful Knowledge Engines: In fields like healthcare, law, or scientific research, RAG can power systems that provide well-informed responses to complex queries.
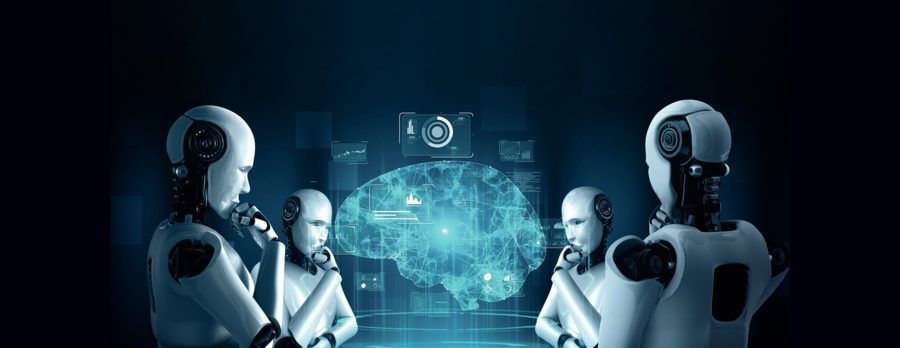
The Future of AI Language Models
As we continue to push the boundaries of what AI can do, techniques like RAG are paving the way for more human-like interactions with AI systems. By addressing common AI challenges such as bias and misinformation, RAG is not just improving the performance of language models – it’s making them more trustworthy and useful in real-world applications.
The potential of RAG is vast, from transforming how we interact with search engines to revolutionizing content creation and knowledge management. As this technology continues to evolve, we can look forward to AI systems that are not just more intelligent, but also more reliable, contextually aware, and truly helpful in our day-to-day lives.
In conclusion, Retrieval-Augmented Generation represents a significant leap forward in AI language model technology. By bridging the gap between vast knowledge sources and generative AI, RAG is setting the stage for a new era of intelligent, informative, and trustworthy AI interactions.
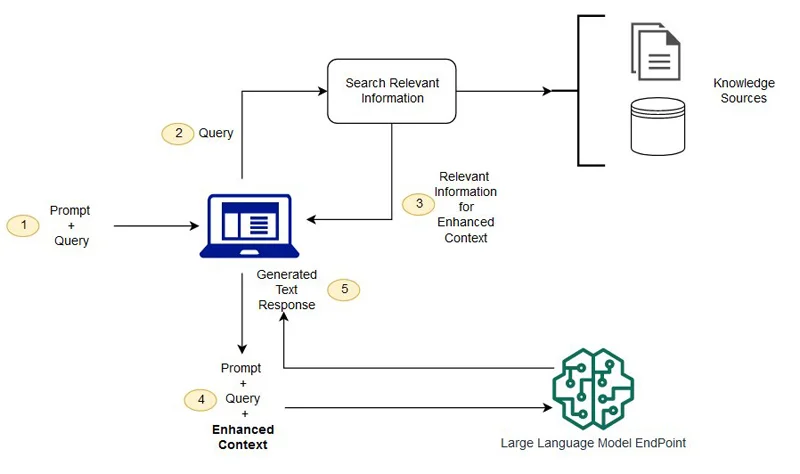